AI Guides
Will AI Replace Software Engineers?
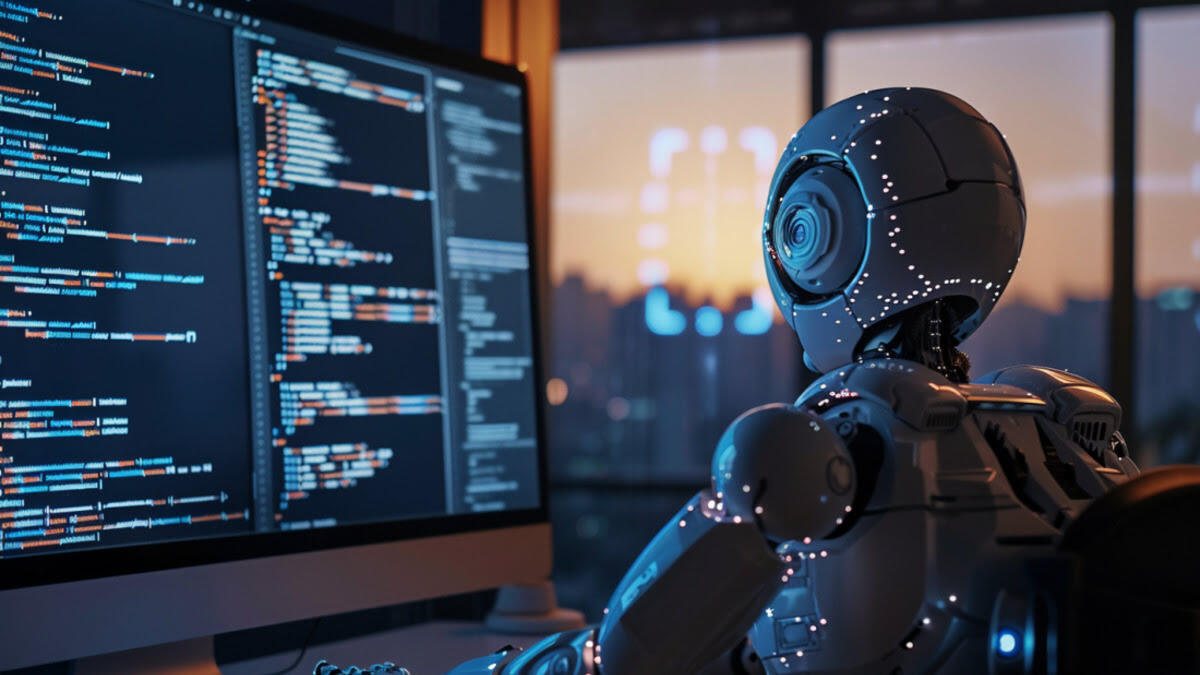
As artificial intelligence (AI) continues to advance, its impact on various professions, including software engineering, is a hot topic of discussion. Among the tools at the forefront of this technological revolution is ChatGPT, a sophisticated AI developed by OpenAI.
With its ability to generate human-like text, ChatGPT raises questions about the future of software engineers: Will it replace them, or become an invaluable tool in their arsenal?
This article will take a deep into how AI like ChatGPT is being integrated into software development and what it means for the future of the profession. We’ll cover the capabilities of AI in automating tasks, enhancing productivity, and the ongoing need for human expertise in the evolving landscape of technology.
The Impact of AI in Software Engineering
Artificial Intelligence (AI) has significantly transformed the field of software engineering, influencing everything from development processes to the roles of the engineers themselves. The integration of AI, particularly tools like ChatGPT, has led to several key advancements and shifts in how software development is approached.
Automation of Routine Tasks
AI has automated many routine and repetitive tasks in software engineering, such as code generation, testing, and debugging. For example, AI-powered tools can analyze written code and suggest improvements or identify errors before they cause problems. This not only speeds up the development process but also enhances the accuracy and efficiency of the final products.
According to GitHub’s Octoverse report, the use of AI in code completion tools like GitHub Copilot has helped reduce the time developers spend writing code by up to 55% (Source: GitHub Octoverse).
Enhanced Code Quality
AI applications in software engineering have also led to improvements in code quality. By utilizing machine learning models that learn from vast datasets of high-quality code, AI tools can provide recommendations that help developers adhere to best practices and coding standards.
Shift in Skill Set Requirements
As AI takes over more routine coding tasks, there is a shift in the skill set required for software engineers. Developers now need to have a strong understanding of AI and machine learning principles to work effectively with these technologies. This shift is reflected in the changing curricula of computer science courses, which increasingly include AI and machine learning components.
Impact on Job Roles
While there is a common fear that AI might replace software engineering jobs, the reality is more nuanced. AI is changing the nature of these jobs but not necessarily reducing the number of them. Software engineers are needed to design, oversee, and integrate AI systems into existing infrastructures.
Limitations of AI in Software Engineering
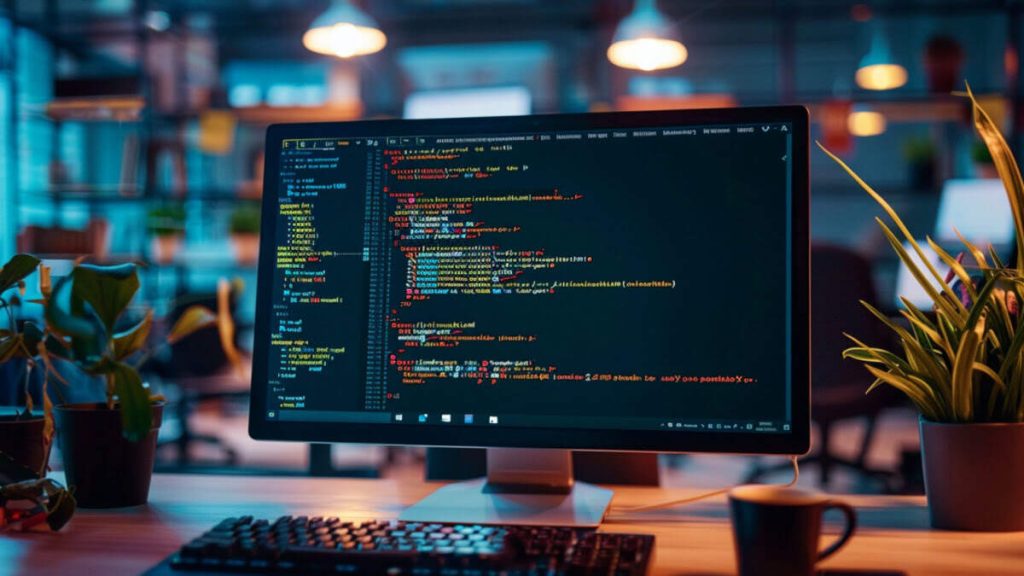
While AI has brought significant advancements to the field of software engineering, it also presents several limitations that can impact its effectiveness and application. Understanding these limitations is crucial for effectively integrating AI into software development processes.
Dependence on Quality Data
AI’s performance is heavily reliant on the quality and quantity of the data it is trained on. In software engineering, this means that AI tools can only be as good as the code and data sets used to train them. Poor quality or biased data can lead to inaccurate suggestions or flawed automation processes.
Lack of Contextual Understanding
AI systems, including advanced language models like ChatGPT, often lack a deep contextual understanding of the projects they are used on. While they can generate code and perform tasks based on patterns, they do not understand the business logic or end goals behind a project. This can lead to recommendations that are technically correct but not suitable for specific project requirements or goals.
Difficulty Handling Complex Problem Solving
AI is excellent for addressing well-defined problems with clear parameters, but it struggles with complex problem-solving that requires high levels of creativity and innovation. Software engineering often involves novel challenges that cannot be easily quantified or solved through standard algorithms. In such cases, human intuition and experience are irreplaceable.
Integration Challenge
Incorporating AI into existing software development workflows can be challenging. Compatibility issues between AI tools and existing software platforms can hinder the seamless integration of automated processes. Additionally, there can be a significant learning curve for teams to effectively utilize AI capabilities, requiring time and resources that may not always be available.
Security and Privacy Concerns
As AI tools become more integrated into software development, they also raise concerns about security and privacy. The use of AI for code generation and automation necessitates access to sensitive project data, which can be a target for cyber threats. Ensuring that AI systems are secure and that data used is protected remains a significant challenge.
What Does All This Mean for Software Engineering Jobs?
As Artificial Intelligence (AI) becomes more embedded in software engineering, it is transforming the profession in profound ways. This evolution is not just about changing the tasks that software engineers perform but also about redefining their roles in the tech ecosystem and the broader job market. Here’s a comprehensive look at how AI is reshaping the career landscape for software engineers:
1) Transformation of Job Roles
AI’s integration into software development is shifting the traditional role of software engineers. As routine coding tasks become automated, the focus shifts towards more complex and strategic work. Software engineers are now expected to manage AI systems, integrate AI with existing infrastructures, and continuously update their skills to keep pace with the advancements in AI technology. This shift is less about displacement and more about evolution, where the core responsibilities are becoming more intellectually demanding and creatively fulfilling.
2) Emergence of New Specializations
The rise of AI is creating new niches and specialties within software engineering. Roles such as AI Ethics Officer, AI System Trainer, and AI Integration Specialist are becoming more common. These roles require a deep understanding of both technical and ethical aspects of AI, blending programming skills with an acute awareness of AI’s broader impacts on society.
3) Increased Demand for AI Literacy
As AI technologies permeate various aspects of business operations, there is a growing demand for software engineers who are not only proficient in traditional programming but also fluent in AI and machine learning concepts. This demand is expanding beyond tech companies to sectors like healthcare, finance, and manufacturing, where AI is used to streamline operations and enhance decision-making processes.
4) Need for Lifelong Learning
The rapid pace of AI development necessitates a commitment to continuous learning and professional development. Software engineers must stay abreast of the latest tools, languages, and AI advancements. Educational institutions and professional development programs are adapting to this change by offering specialized courses in AI and machine learning, which are becoming essential for upcoming software engineers.
5) Broader Implications for Employment
While AI introduces new tools and capabilities in software engineering, it also brings challenges, particularly in terms of job displacement in lower-level coding positions. However, this displacement is also accompanied by job creation, particularly in areas that require high-level analytical and managerial skills. The net effect of AI on software engineering employment is complex and multifaceted, suggesting a shift in the types of jobs available rather than a straightforward reduction in job opportunities.
6) Global Impact and Accessibility
AI is democratizing the field of software development by enabling more people to build software through AI-driven code generation tools, lowering the barrier to entry for software development. This has the potential to globalize the field further, spreading job opportunities across different regions and reducing the concentration of jobs in certain tech hubs.
7) Ethical and Societal Considerations:
As AI reshapes the software engineering landscape, it also raises ethical questions that professionals in the field must address. This includes issues like bias in AI algorithms, privacy concerns, and the societal impact of automated systems. Addressing these issues responsibly is becoming a part of the job description for software engineers.
No, AI Won’t Replace Software Engineers
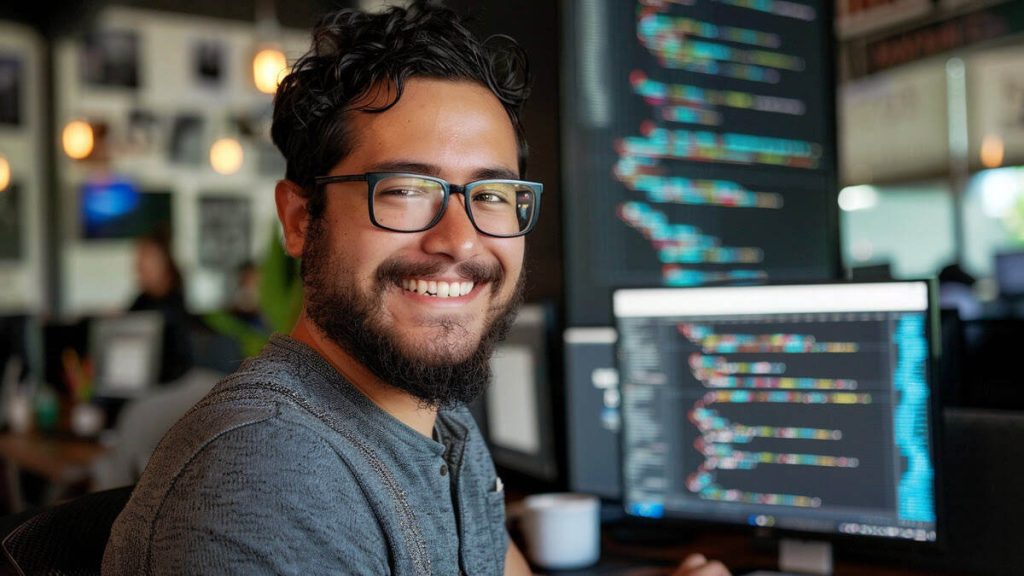
As we’ve explored throughout this article, the integration of Artificial Intelligence (AI) into software engineering is undoubtedly reshaping the landscape of the profession. AI has proven its capability to automate routine tasks, enhance productivity, and even tackle complex problem-solving with efficiency. However, despite these advancements, AI is not poised to replace software engineers in the foreseeable future.
AI excels at specific tasks where patterns and data are abundant, but it lacks the nuanced understanding and creative problem-solving inherent to human intelligence. Software engineers bring critical thinking, strategic insight, and ethical considerations to projects—qualities that AI cannot replicate. Rather than replacing engineers, AI is transforming the role, enabling them to focus on more innovative and complex aspects of software development.
The evolution in the field calls for software engineers to adapt by enhancing their skills in AI and machine learning. This adaptation is not just about staying relevant but also about leveraging AI to create more sophisticated, efficient, and impactful software solutions. As AI continues to develop, it will serve as a powerful tool in the engineer’s toolkit, augmenting human capabilities rather than supplanting them.
In summary, the relationship between AI and software engineering is symbiotic. AI may change how engineers work, but it also opens up new opportunities for innovation and efficiency. The future of software engineering will likely see AI and human intelligence working in tandem, each complementing the other to push the boundaries of what’s possible in technology and beyond.
AI Guides
History of AI – From the 1950s to Present
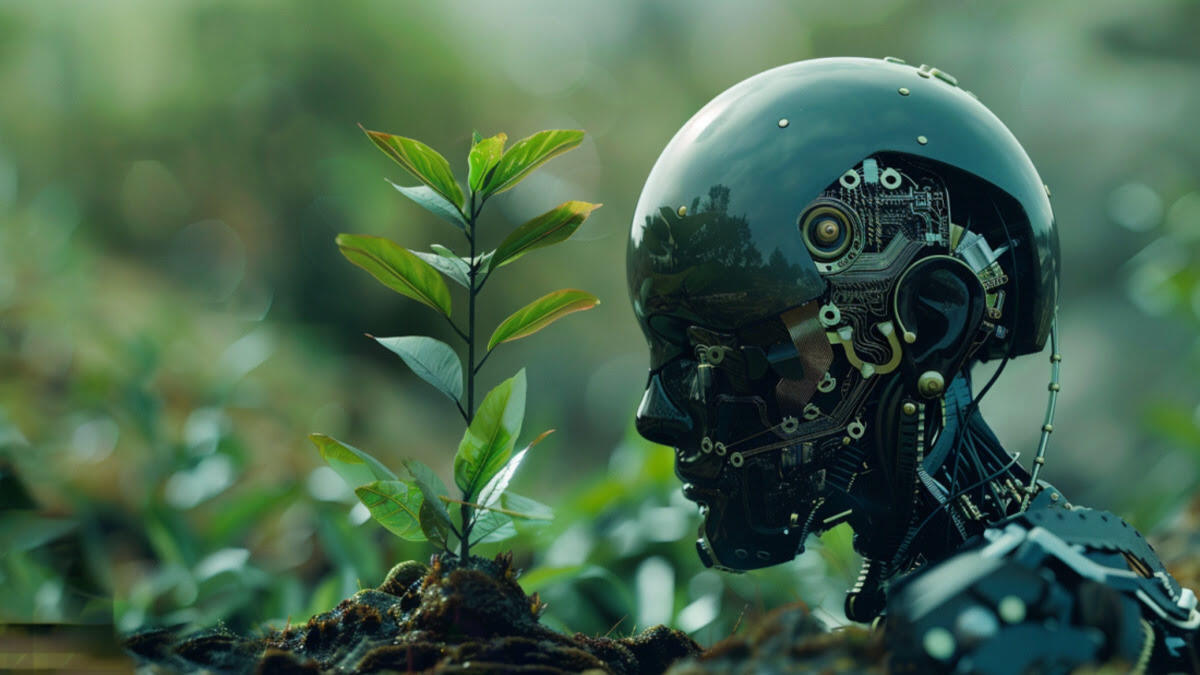
Artificial Intelligence (AI) might seem like a concept straight out of a modern sci-fi movie that entered our lives in the past couple of years, but did you know that the idea has been around for centuries?
In this article, we’ll dive into the history of AI, tracing its origins and major milestones. Continue reading to the end to discover how AI has evolved through history.
The Early Imaginings and Theoretical Foundations
Long before the term “artificial intelligence” was coined, humans dreamed of creating intelligent machines. Ancient myths and stories from various cultures feature mechanical beings endowed with intelligence, showcasing early human fascination with mimicking life through machinery. For instance, in Greek mythology, Hephaestus, the god of craftsmanship, created mechanical servants.
Fast forward to the 17th and 18th centuries during the Enlightenment, when philosophers and mathematicians like René Descartes and Gottfried Wilhelm Leibniz began pondering the possibility of machines thinking like humans. They discussed the human mind in terms of a complex machine, laying the groundwork for computational thinking.
The Formal Birth of AI (1950s – 1960s)
The actual term “Artificial Intelligence” was first used in 1956 by John McCarthy, a young assistant professor at Dartmouth College, who organized a pivotal conference – now considered the birth of AI as a field of study.
This event, known as the Dartmouth Summer Research Project on Artificial Intelligence, brought together researchers interested in neural networks, the study of intelligence, and the possibility of replicating human thought in machines.
During this era, AI research received significant attention and funding. Early successes included programs that could perform algebraic equations and play checkers at a high level. These developments led to optimistic predictions about AI’s future, setting high expectations for rapid progress.
The First AI Winter (1970s)
Despite early enthusiasm, progress in AI research did not keep pace with expectations. By the mid-1970s, the limitations of existing AI technology became apparent, leading to the first AI Winter, a period marked by reduced funding and waning interest in AI research. This downturn was largely due to the overly ambitious expectations that could not be met by the technology of the time, which struggled with real-world applications.
The Resurgence and Rise of Machine Learning (1980s – 1990s)
AI experienced a resurgence in the 1980s, thanks in part to the adoption of machine learning. Instead of trying to directly encode AI with extensive knowledge and rules about the world, researchers focused on creating algorithms that could learn from data.
This shift was significant, leading to more robust and adaptable AI systems. The introduction of backpropagation by researchers such as Geoffrey Hinton allowed neural networks to learn from their errors, improving their performance over time.
During this period, governments and industries began investing heavily in AI again, intrigued by its potential applications. AI started to be used for logistics, data management, and within expert systems in fields like medicine and engineering, marking its transition from a purely academic pursuit to a practical tool in business and other areas.
By the late 1990s, the internet boom provided AI researchers with unprecedented amounts of data and a new platform to deploy AI technologies. This period led to significant advancements in algorithms and the capability of AI systems to handle tasks involving big data, marking another turning point in the AI development timeline.
As we continue exploring the evolution of AI, we will see how the 21st century brought AI into our daily lives, making it an indispensable tool in various sectors, from healthcare to entertainment. Stay tuned as we uncover more about how AI continues to evolve and shape our world in ways we could hardly imagine just a few decades ago.
AI in the 21st Century: Expansion into Daily Life and Beyond
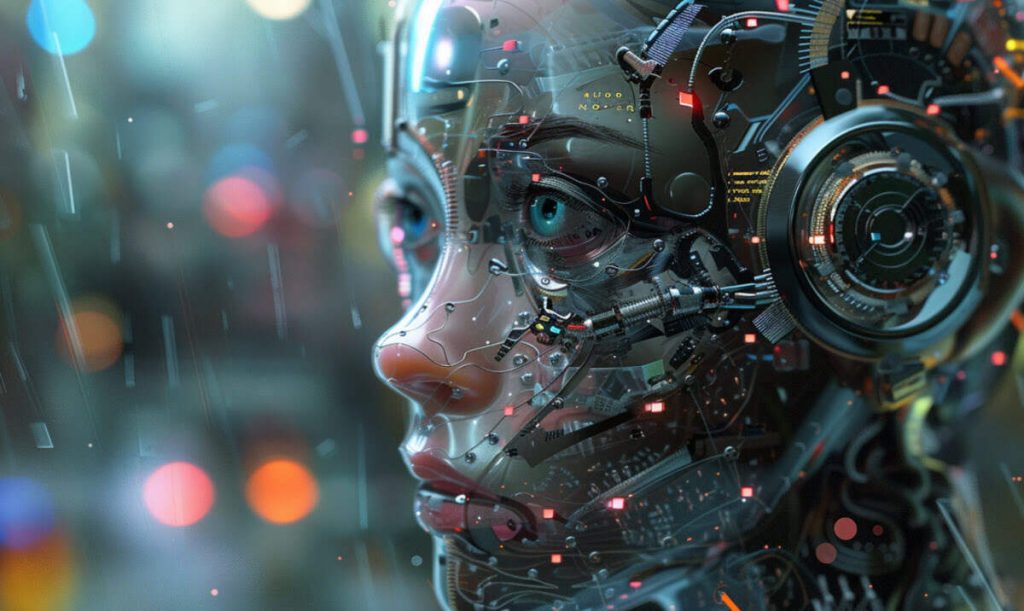
As the new millennium unfolded, AI’s integration into daily life and various sectors accelerated at an unprecedented pace. The development of sophisticated machine learning models, particularly Deep Learning, has enabled AI to analyze and generate large volumes of data with astonishing accuracy.
This section of our journey through the history of artificial intelligence will explore how AI has become a ubiquitous part of modern life.
Deep Learning and Big Data
The 2000s witnessed a major breakthrough with the advent of deep learning techniques, which involve neural networks with many layers that can learn increasingly abstract features of data. These networks were fueled by the explosive growth of “big data” generated by the digital activities of businesses and consumers alike.
Companies like Google, Amazon, and Facebook began using deep learning to improve products and services, from enhancing search algorithms to personalizing advertisements, thereby making AI an integral part of the tech industry’s infrastructure.
AI in Consumer Technology
Perhaps the most relatable example of AI for most people is its role in consumer technology. Virtual assistants like Apple’s Siri, Amazon’s Alexa, and Google Assistant use AI to understand and respond to voice commands, providing users with information, entertainment, and assistance with daily tasks.
The seamless integration of AI into smartphones and home devices has dramatically changed how people interact with technology, making AI a helpful companion in our everyday lives.
Autonomous Vehicles
Another significant area of AI development is in autonomous vehicles. Companies like Tesla, Waymo, and Uber have invested heavily in AI systems that can safely navigate roads without human intervention. These vehicles use AI to process inputs from various sensors and cameras, making split-second decisions that can adapt to complex traffic environments and driving conditions.
AI in Healthcare
AI’s impact on healthcare has been profound, offering tools for diagnosis, personalized medicine, and patient management. AI algorithms can analyze medical images with accuracy that matches or exceeds human radiologists.
Additionally, AI is used to predict patient outcomes, personalize treatment plans, and manage healthcare records more efficiently, significantly improving the quality of care and operational efficiencies in healthcare facilities.
How AI Continues to Shape Our Future
The journey of AI from a concept in myths to a key player in major industries shows its vast potential and inevitable growth. As AI technology continues to evolve, its capabilities will likely become more sophisticated, leading to even more innovative applications across different sectors.
Ethical Considerations and Future Challenges
However, the rapid growth of AI also brings challenges, particularly ethical considerations like privacy, security, and the impact of automation on employment. The future of AI will likely focus not only on technological advancements but also on addressing these ethical issues, ensuring that AI benefits society as a whole.
The Road Ahead
Looking forward, the integration of AI in more complex tasks and its potential to understand human emotions and make morally significant decisions are areas of intense research and interest. The journey of AI is far from over; it is evolving every day, promising a future where AI and humans coexist, complementing each other’s capabilities.
Conclusion
The history of artificial intelligence is a fascinating tale of human ingenuity and technological advancement. From early automata to sophisticated AI that permeates every aspect of our lives, AI’s journey is a testament to the relentless pursuit of knowledge and understanding by scientists, engineers, and thinkers across generations.
As we stand on the shoulders of these pioneers, we look forward to a future where AI continues to enhance our abilities and enrich our lives.
AI Guides
Why Can’t AI Art Make Hands
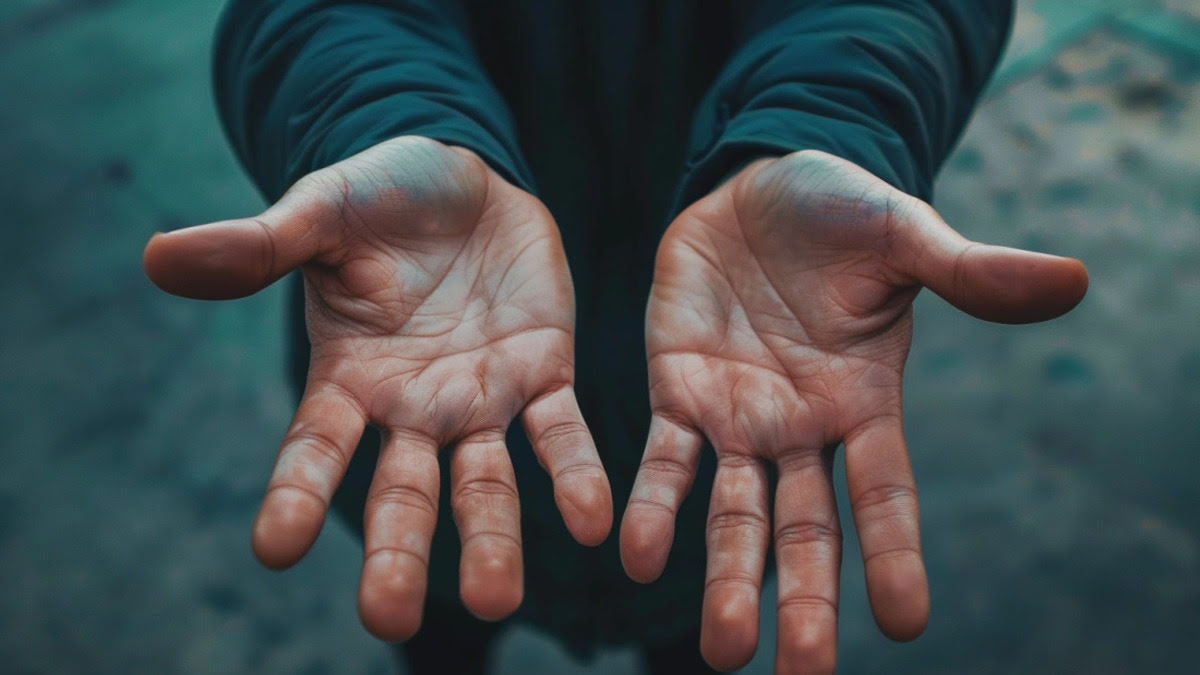
Artificial Intelligence (AI) has made significant strides in many fields, and art creation is no exception. AI art generators, like those powered by machine learning models such as DALL-E or GANs (Generative Adversarial Networks), can create stunning images that dazzle the imagination.
These tools are used for everything from generating abstract art for digital spaces to crafting backgrounds for games and virtual realities. Despite their capabilities, these AI systems often struggle with a peculiar challenge: drawing human hands accurately.
Our article will explain why AI art generators frequently produce hands that look awkward, distorted, or downright eerie, and why hands are a particularly tough challenge for AI. Read on to know why AI finds hands so difficult to get right, and why this matters more than you think for the future of AI-generated art.
Why AI Struggles With Generating Human Hands
Human hands are one of the most complex and detailed parts of the body, involving a wide range of motions and configurations that can express a multitude of gestures and actions. This complexity presents a significant challenge for AI image generators, not just for one but for several reasons.
Below, you’ll find why AI art generators struggle with drawing hands.
High Variability
Hands are highly variable in their appearance and position. They can interact with numerous objects, appear in countless poses, and each hand gesture can convey different emotions or actions.
For AI, which learns from a dataset of existing images, the immense variability of hand positions and their interactions with other objects can lead to a lack of comprehensive learning material. As a result, the AI often struggles to accurately recreate hand positions that it hasn’t encountered frequently in its training set.
Intricate Detailing
The structure of a hand is intricate, with fine detailing in the knuckles, nails, and skin texture. Each of these details needs to be rendered accurately for a hand to look realistic.
AI systems typically generate images based on patterns they have learned from data; if the details in the training images are not diverse or detailed enough, the AI will have difficulty replicating them accurately. This often results in hands that look flat, malformed, or overly simplified.
Complex Interactions
Hands are rarely seen in isolation; they are usually interacting with objects or other parts of the body. This interaction adds a layer of complexity to the image generation process.
AI must not only generate the hand but also understand and replicate how it interacts with its environment. This requires an understanding of physics, space, and object dynamics, which are challenging for AI to learn completely.
Data Limitations
The quality of the data used to train AI significantly impacts its output. If the dataset is not diverse enough or lacks high-quality images of hands in various poses and interactions, the AI will struggle to generate high-quality images of hands.
Moreover, biased or insufficient training data can lead to repetitive errors, such as consistently generating an incorrect number of fingers or unrealistic hand shapes.
Other Parts of the Human Body AI Struggles to Generate
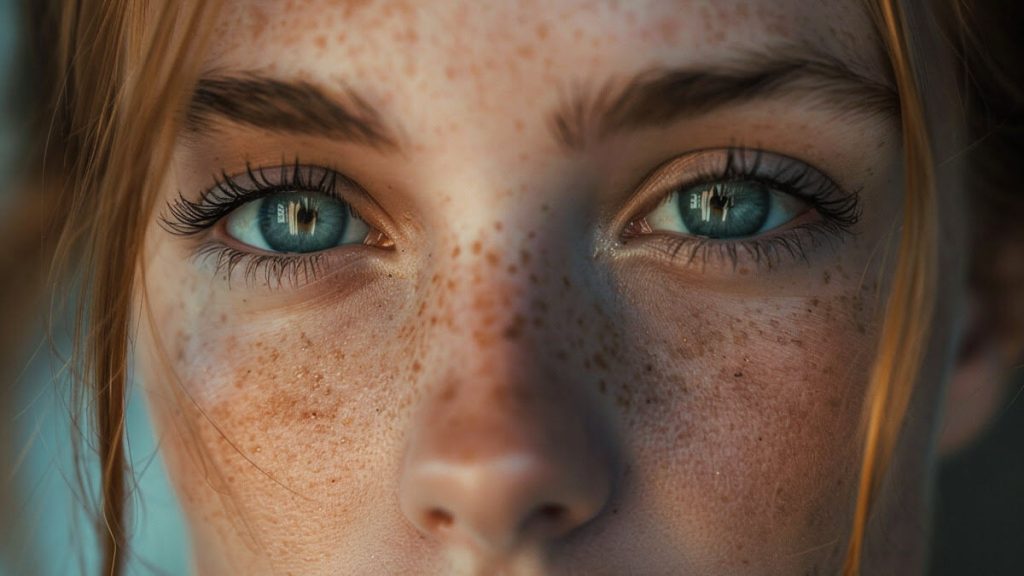
While AI’s difficulties with generating realistic human hands are well-documented, this challenge extends to other complex parts of the human body as well. Features such as faces, feet, and hair also present significant hurdles for AI image generators.
The reasons for these struggles often overlap with some of those seen in hand generation. Let’s explore why AI particularly struggles with these features.
Faces
The human face is a centerpiece of identity and expression, involving subtle micro-expressions that convey a wide range of emotions, from joy to sorrow. AI often struggles to replicate these nuances for several reasons:
- Complexity of Expressions: Human expressions involve small, often rapid changes in facial muscles. AI systems find it challenging to capture these nuances accurately because they require an understanding of how muscles interact and how expressions change dynamically over time.
- Symmetry and Proportions: Human faces have a specific symmetry and proportion that can be difficult for AI to replicate accurately. Even slight deviations in symmetry or proportions can make a face look unnatural or unsettling.
- Eye Detailing: The eyes are particularly expressive and detailed parts of the face. AI systems often struggle to render the depth and sparkle of human eyes, which are critical for a face to appear lifelike and relatable.
Feet
Like hands, feet are complex structures that involve many small bones, joints, and types of movements. AI struggles with feet for similar reasons:
- Variability in Position: Feet can appear in numerous positions depending on the body’s actions, such as standing, running, or resting. Capturing these positions accurately, along with the associated shadows and textures, is challenging for AI.
- Interaction with Surfaces: Feet often interact with various surfaces, which can affect their appearance. AI must understand and replicate these interactions, such as the flattening of the soles when standing or the arching of the toes when walking, which is a complex task.
Hair
Hair presents another significant challenge for AI due to its fluid and dynamic nature:
- Texture and Flow: Hair has different textures and styles that can change with movement and environmental conditions, such as wind or humidity. AI systems often struggle to generate hair that looks natural and flows realistically.
- Volume and Light Interaction: Accurately rendering how hair volumes interact with light and shadow is complex. Hair also has varying degrees of transparency and reflectivity, which are difficult for AI to replicate, often resulting in hair that looks either too heavy or too light.
All of these features require a deep understanding of human anatomy, the physics of light and materials, and the subtleties of human expression, all of which are areas where AI still has room for improvement.
As AI technology evolves, the ability to handle these complex human features with greater accuracy will continue to grow, driven by advances in machine learning models, increased computational power, and more extensive training datasets.
These improvements will help AI overcome its current limitations, allowing for more realistic and nuanced representations of human features in digital art and other applications.
How to Help AI Get Human Features Right
If you’re using AI and tired of it not getting parts of the human body right, there are a few things you can do to fix this – or at least make it easier for the AI to generate better-looking images.
Here are several practical steps that can help improve the accuracy of AI-generated human features:
Use High-Quality, Detailed Images
The quality of images used in training datasets significantly impacts AI’s output. High-resolution images that show detailed features of hands, facial expressions, and interactions can provide the AI with a better understanding of subtle details. This is particularly crucial for intricate parts like the texturing of skin, the way light plays on muscle, or the specifics of hand positioning.
Implement Advanced Modeling Techniques
Employing advanced neural network models that focus on depth and texture can aid in generating more realistic human features. Techniques such as Generative Adversarial Networks (GANs) have been particularly successful in creating photorealistic images. These models learn to simulate fine details more accurately by pitting two neural networks against each other: one generates images; the other evaluates their realism.
Community Feedback
The AI development community can be a tremendous resource. Platforms like Reddit often feature discussions where users share their experiences with different AI tools, providing insights into common issues and potential solutions. By engaging with these communities, you can find solutions to your common problems with AI-generated images.
AI Is Getting Better at Generating Images Every Day
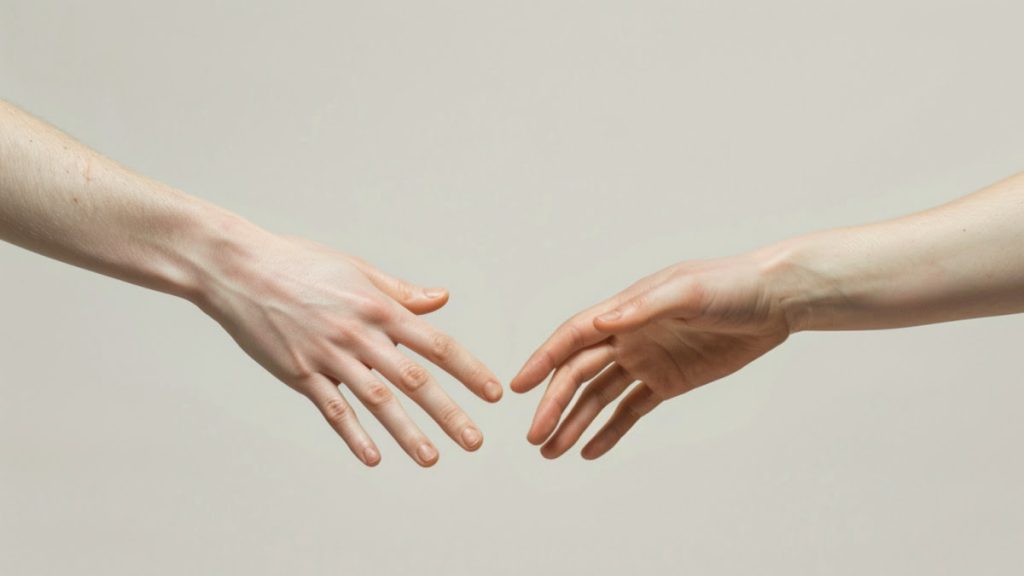
Despite the current challenges, AI technology is improving rapidly, and the quality of images it can generate is getting better every day. Developers are continually working on refining AI algorithms, expanding training datasets, and incorporating user feedback into the development process. These efforts are gradually overcoming the difficulties AI faces with complex human features like hands, faces, and hair.
Several AI tools are already making significant strides in this area. For instance, newer versions of AI image generators have begun to show improved capability in handling human anatomy with greater accuracy. These advancements suggest a promising future where AI can not only match but potentially exceed human capabilities in creating detailed, realistic images.
As AI continues to evolve, it holds the potential to transform artistic creation, offering tools that augment human creativity with digital precision. For artists, designers, and creators, these developments signal exciting new possibilities for collaboration between human imagination and AI efficiency, opening up a world of creative opportunities that were once thought impossible.
AI Guides
GPT-4 vs. GPT-4o: Key Differences
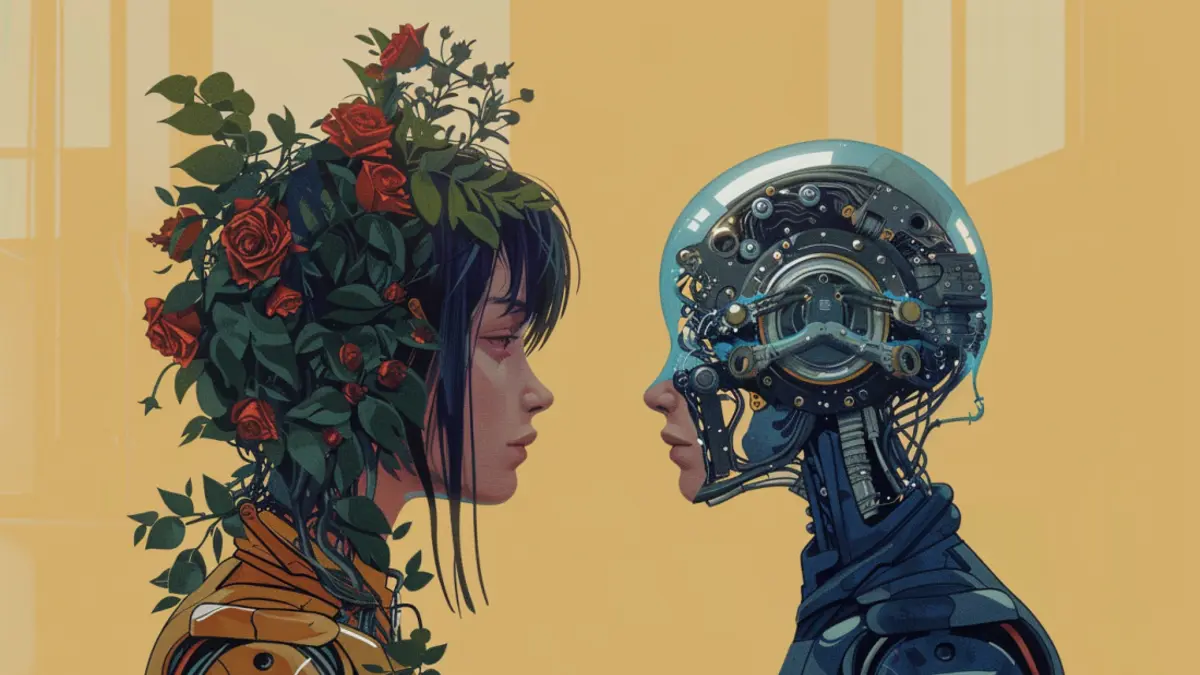
GPT-4 is a powerful AI language model developed by OpenAI. It excels at understanding and generating human-like text. GPT-4o is the latest version, introducing more advanced features, making interactions more natural and dynamic. This comparison explores the differences and improvements brought by GPT-4o.
GPT-4o enhances the capabilities of GPT-4 by integrating voice and video inputs, which allows for more interactive and versatile applications. This new model aims to provide a more immersive user experience, extending beyond text-based interactions.
Our article will go over nine key differences between the GPT-4 and GPT-4o that you should know. Read to the end to know these differences to determine which one suits your needs the most.
9 Key Differences Between GPT-4 and GPT-4o
Development and Release Timeline
GPT-4 was released earlier, gaining popularity for its advanced text processing. OpenAI then developed GPT-4o to build on this success. GPT-4o was officially released in May 2024, marking a significant advancement in AI technology.
The development of GPT-4o focused on adding new features and improving speed and responsiveness. This upgrade aims to make the AI more efficient and versatile, capable of handling more complex tasks in real-time.
Core Functionalities
GPT-4 is known for its strong capabilities in natural language understanding and generation. It can perform tasks such as answering questions, writing essays, and generating creative content. It is used in various applications like chatbots, content creation, and coding assistance.
GPT-4o retains these functionalities but expands on them with voice and video integration. This means GPT-4o can understand and generate responses not just in text but also through spoken words and visual inputs, making it a more comprehensive AI model.
Speed and Responsiveness
GPT-4 is already fast, but GPT-4o takes responsiveness to a new level. GPT-4o can respond to voice commands in about 232 milliseconds, which is nearly as fast as human reaction time. This speed enhances the feeling of a natural conversation.
The increased speed and reduced latency in GPT-4o make it more suitable for applications requiring real-time interaction, such as virtual assistants and customer service. This improvement helps in making interactions smoother and more efficient.
Multimodal Capabilities
GPT-4 focuses on text-based inputs and outputs, excelling in generating high-quality text and understanding complex language inputs. This makes it suitable for applications that rely solely on text, such as chatbots, writing assistants, and text analysis tools.
In contrast, GPT-4o is a multimodal model, meaning it can process and generate text, voice, and video inputs. This allows GPT-4o to handle a broader range of tasks, such as real-time video analysis, voice-based interactions, and text responses. This makes it a versatile tool for various applications beyond text, such as virtual assistants and interactive learning environments.
Voice and Video Integration
One of the most significant advancements in GPT-4o is its integration of voice and video capabilities. Users can now interact with the AI using their voice, and the AI can respond with natural-sounding speech. Additionally, GPT-4o can process video inputs, making it capable of understanding and analyzing visual content in real-time.
This integration allows GPT-4o to be used in more engaging ways. For example, it can act as a virtual tutor, providing explanations and showing videos to illustrate points. It can also assist in customer service by offering spoken responses and understanding visual queries, enhancing the user experience significantly.
Real-Time Interaction
GPT-4o excels in real-time interaction, handling dynamic conversations more effectively than GPT-4. It can manage interruptions and quickly adjust to changes in the conversation, providing a more fluid and natural interaction experience. This makes interactions feel more like a natural conversation with a human.
This real-time capability is particularly useful for applications like live customer support and interactive personal assistants. GPT-4o can maintain the flow of conversation without delays, enhancing user experience and communication efficiency. Its ability to respond quickly and handle conversational changes makes it a valuable tool in dynamic environments.
Vision and Image Processing
While GPT-4 is limited to text, GPT-4o includes advanced vision capabilities. It can analyze images and videos, understand handwritten text, and solve visual problems in real-time. This feature significantly expands the range of applications for GPT-4o.
For example, GPT-4o can assist with homework by analyzing handwritten notes or solving math problems shown through a phone’s camera. It can also provide detailed descriptions of images and videos, making it useful for visual content analysis and educational purposes. This visual capability sets GPT-4o apart from GPT-4, providing more comprehensive assistance in various tasks.
Use Cases and Applications
GPT-4 has been widely used in chatbots, content creation, and coding assistance. Its text-based capabilities make it suitable for a variety of applications where natural language processing is needed. Many companies use GPT-4 to enhance their services and products, benefiting from its robust text generation and understanding abilities.
GPT-4o extends these applications by incorporating voice and video features. It can be used as a virtual tutor, offering explanations and showing videos. In customer service, it provides quick and natural interactions with spoken responses and visual understanding. GPT-4o’s multimodal capabilities make it a more versatile tool for different tasks, expanding its usability beyond text-based applications.
User Accessibility and Availability
GPT-4 is available through various platforms, and developers can access its API to build applications. It has been widely adopted by users for different tasks, making it a popular choice for AI solutions. Many people use GPT-4 every day for various purposes, from casual conversations to professional tasks.
GPT-4o is also accessible to all users, including those on the free tier of ChatGPT. This wider availability ensures that more people can experience its advanced capabilities. OpenAI is gradually rolling out GPT-4o’s new features, making sure the transition is smooth and that users can easily adapt to the new model. This accessibility allows a broader audience to benefit from GPT-4o’s advanced functionalities.
Future ChatGPT Updates
The release of GPT-4o demonstrates the rapid advancement of AI technology. OpenAI continues to improve its models, adding new features and enhancing performance. Future updates to GPT-4o will likely introduce even more capabilities, making AI an integral part of everyday life.
Keeping up with these developments is important as AI technology evolves. GPT-4o represents just the beginning of what advanced AI models can achieve. The future promises more intelligent and helpful AI tools that will continue to transform various aspects of our lives. Users can look forward to even smarter and more versatile AI solutions in the near future.
-
AI Guides1 year ago
GPT-4 vs. GPT-4o: Key Differences
-
AI for Investment1 year ago
How to Use AI for Stock Trading
-
AI Guides1 year ago
History of AI – From the 1950s to Present
-
AI Guides1 year ago
Current Limitations of Artificial Intelligence
-
AI Tool Reviews1 year ago
10 Best Free AI Websites
-
AI for Entertainment1 year ago
AI Predicts for Super Bowl Winners 2024 to 2099 – Surprising Results
-
AI Tool Reviews1 year ago
Perplexity AI Review: Better Than ChatGPT?
-
AI Tool Reviews1 year ago
10 Best AI Song Generators (Including Free Options)